Healthcare is experiencing a seismic shift, and artificial intelligence is the force behind it. No longer confined to science fiction, AI systems are already working alongside doctors, analyzing test results, and helping discover new medications. But what makes this technology so powerful in medicine is its ability to find patterns in massive datasets that humans simply can’t process alone.
I’ve been tracking AI development across industries for years, and healthcare stands out as the field with perhaps the most profound potential impact. When algorithms can detect cancer earlier than human eyes or predict patient deterioration before traditional monitors show warning signs, we’re entering new territory in medicine. And this is just the beginning.
The integration of AI into healthcare represents more than just technological advancement—it’s fundamentally changing the relationship between providers and patients, researchers and diseases, and even how we think about wellness itself.
AI in Healthcare: The Transformation Landscape
Healthcare has traditionally moved cautiously with new technologies, and for good reason—lives are at stake. But AI adoption is accelerating rapidly as the benefits become impossible to ignore. The global AI in healthcare market is projected to reach $187.95 billion by 2030, up from approximately $11 billion in 2021.
What’s driving this growth? For one, the explosion of medical data. From electronic health records to medical imaging to genomic sequencing, healthcare now generates exabytes of information that traditional analysis simply cannot handle efficiently. AI thrives on exactly this kind of data abundance.
The COVID-19 pandemic also accelerated adoption, demonstrating how quickly AI could help track disease spread, identify potential treatments, and even speed vaccine development. This real-world proof of concept helped overcome institutional resistance to AI implementation that had previously slowed progress.
Perhaps most importantly, AI is addressing critical healthcare challenges—physician burnout, diagnostic errors, healthcare disparities, and the growing needs of an aging population. These pressures have created fertile ground for technological solutions that can scale expertise and extend the reach of medical professionals.
Key Areas Where AI is Revolutionizing Medicine
Superhuman Diagnostics
If there’s one area where AI is already outperforming humans, it’s in certain diagnostic tasks. AI systems can now detect diabetic retinopathy, skin cancers, and lung nodules with accuracy rates that match or exceed specialized physicians. This isn’t about replacing doctors but augmenting their capabilities.
For example, a study published in the National Library of Medicine showed that AI systems could identify early signs of breast cancer in mammograms that radiologists missed, potentially saving lives through earlier intervention. The algorithm found subtle patterns in tissue density and calcification that even experienced specialists couldn’t consistently identify.
What makes these diagnostic tools particularly valuable is their consistency—they don’t get tired, rushed, or distracted. They apply the same meticulous analysis to each case, whether it’s the first patient of the day or the fiftieth.
I’ve spoken with radiologists who initially feared AI would make their profession obsolete. Now many are embracing these tools because they handle routine cases while allowing specialists to focus on complex diagnoses where human expertise truly adds value. It’s becoming a powerful partnership rather than a replacement scenario.
Personalized Treatment Pathways
Healthcare has long aspired to move beyond one-size-fits-all treatments, but individual variability in patient response made true personalization difficult. AI is changing that equation by analyzing thousands of variables simultaneously.
These systems can integrate genetic information, medical history, lifestyle factors, and even social determinants of health to recommend treatments with the highest likelihood of success for a specific patient. The move from population-based medicine to precision healthcare is happening right now.
One fascinating application is in cancer treatment, where AI platforms analyze tumor genomics and compare them against databases of treatment outcomes to suggest the most effective therapy options. According to healthcare AI specialists, this approach is reducing trial-and-error treatment attempts and helping patients receive effective interventions sooner.
What’s particularly exciting about personalized medicine through AI is how it democratizes expertise. A patient in a rural clinic can potentially receive treatment recommendations informed by outcomes from leading medical centers around the world, narrowing the gap in care quality.
Accelerated Drug Discovery and Development
The traditional drug development timeline—often 10+ years and billions of dollars—is being dramatically compressed through AI applications. Machine learning algorithms can predict how molecular compounds will behave in the body, significantly reducing the need for early-stage trial-and-error testing.
We’re seeing AI platforms that can:
- Screen billions of potential compounds in days rather than years
- Predict drug interactions and side effects before clinical trials
- Identify existing medications that could be repurposed for new conditions
- Design novel molecules specifically targeted to disease mechanisms
During the COVID-19 pandemic, AI systems identified potential treatments within weeks of receiving viral genome data. This rapid response capability represents a fundamental shift in how we approach emerging health threats.
Companies using AI tools for business operations in the pharmaceutical sector are reporting 50-60% reductions in early-stage development timelines. This acceleration means more treatments reaching patients faster and potentially at lower costs.
Automated Patient Monitoring and Care
AI-powered monitoring systems are transforming both acute and chronic care management. In hospital settings, algorithms continuously analyze vital signs and lab values to detect subtle patterns that precede deterioration, often hours before traditional monitoring would trigger alerts.
For chronic conditions, remote monitoring with AI analysis enables healthcare providers to track patients between appointments. These systems can:
- Detect medication non-adherence through behavioral patterns
- Identify environmental factors affecting condition management
- Predict exacerbations of conditions like COPD or heart failure
- Recommend preventive interventions before emergency care is needed
The impact extends to mental health as well, with AI-powered communication tools that can detect linguistic patterns associated with depression, anxiety, or suicidal ideation, creating opportunities for early intervention.
What I find particularly valuable about these monitoring systems is how they extend the reach of healthcare beyond traditional settings. Patients can receive meaningful oversight at home, work, or while traveling—truly continuous care rather than episodic medical encounters.
Operational Intelligence for Healthcare Systems
Beyond direct patient care, AI is transforming how healthcare organizations function. Predictive models now forecast patient admission volumes, allowing hospitals to staff appropriately and reduce wait times. Resource allocation algorithms ensure critical supplies are available when and where needed.
Administrative applications are equally powerful. Natural language processing extracts relevant information from clinical notes, reducing documentation burden for providers. Automated coding systems ensure accurate billing while freeing staff for more valuable activities.
These operational improvements might seem less glamorous than diagnostic breakthroughs, but their impact on healthcare quality, access, and affordability is profound. When Harvard researchers studied AI implementation in health systems, they found operational applications often delivered the most immediate financial and quality benefits.
Practical Applications and Future Directions
For healthcare professionals considering how to integrate AI into their practice, there are several entry points worth considering:
Start with specific, well-defined use cases where AI has demonstrated clear benefits, such as image analysis or predictive analytics for high-risk patients. These applications usually have significant research validation and established implementation pathways.
Invest in data infrastructure before AI implementation. Clean, structured data is the foundation of effective AI, and many healthcare organizations find their initial challenge is not algorithm selection but data preparation and integration.
Remember that AI implementation is as much about workflow redesign as technology adoption. The most successful projects carefully consider how AI tools integrate into clinical processes and provide adequate training for all staff members who will interact with these systems.
For those looking to build expertise in this field, specialized AI in healthcare courses are now available through major learning platforms. These programs range from introductory overviews to technical deep dives on algorithm development.
Looking ahead, several emerging applications show particular promise:
- Digital twins that simulate individual patient physiology for treatment testing
- Multimodal AI systems that integrate data from wearables, genomics, and clinical records
- Ambient clinical intelligence that automatically documents patient encounters
- Edge computing devices that bring advanced diagnostics to remote locations
If you’re considering AI implementation for your healthcare organization, TechMim’s integration consultations can help identify the highest-value applications for your specific needs and develop implementation roadmaps that maximize return while minimizing disruption.
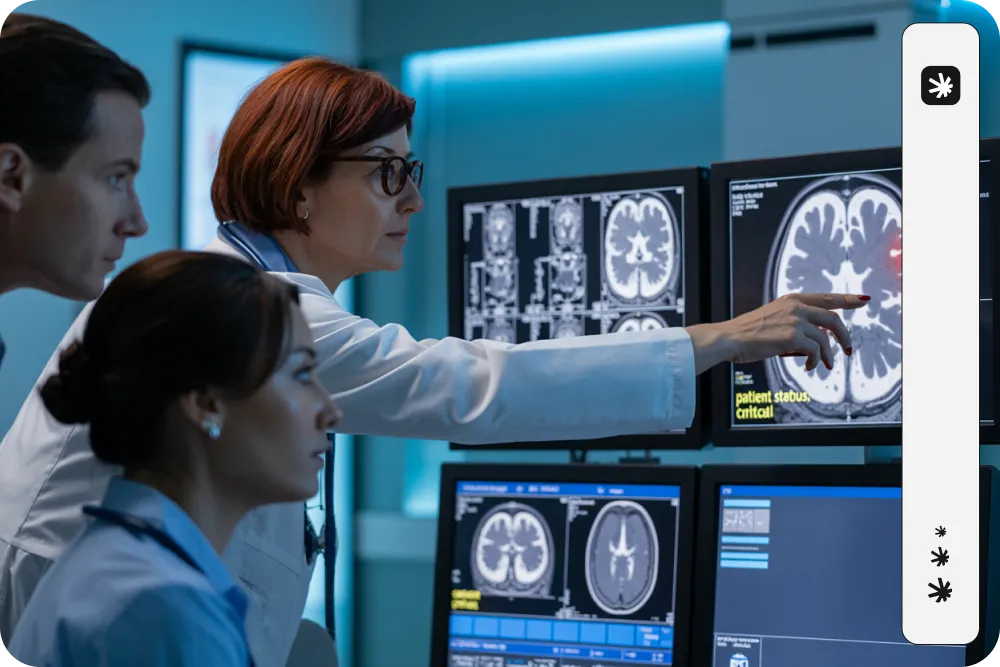
Navigating Challenges in Healthcare AI
Despite tremendous promise, AI in healthcare faces significant challenges that require thoughtful navigation:
Data Privacy and Security
Healthcare data is uniquely sensitive, making privacy concerns particularly acute. Robust anonymization techniques and secure computing environments are essential for maintaining patient trust. Current regulations like HIPAA weren’t designed with AI applications in mind, creating compliance uncertainties.
Several promising approaches are emerging, including federated learning (where algorithms travel to data rather than centralizing sensitive information) and differential privacy techniques that enable analysis while mathematically guaranteeing individual privacy.
Algorithmic Bias and Healthcare Disparities
AI systems can inadvertently perpetuate or amplify existing healthcare disparities if trained on non-representative data. For instance, algorithms trained primarily on data from one demographic group may perform poorly for others, potentially worsening rather than reducing care gaps.
Industry consultants recommend diverse development teams, explicit bias testing, and ongoing performance monitoring across different patient populations. The goal isn’t perfect algorithms but ones whose limitations are well-understood and mitigated.
Clinical Integration and Workflow Disruption
Even the most accurate AI tools deliver no value if they disrupt clinical workflows or create additional burden for healthcare providers. Successful implementation requires careful attention to user experience design and workflow integration.
AI deployment should reduce cognitive load rather than adding to it. This means thoughtful interface design, appropriate automation of routine tasks, and intelligent notification systems that provide actionable insights without overwhelming users.
Regulatory Frameworks and Liability
Regulatory frameworks for AI in healthcare are still evolving, creating uncertainty about approval pathways and liability in case of errors. Who bears responsibility when an AI system contributes to a medical error—the developer, the healthcare facility, the clinician who used the recommendation?
These questions don’t have simple answers, but organizations leading in healthcare AI are actively engaging with regulatory bodies to help shape frameworks that ensure safety while enabling innovation.
Conclusion: The Human-AI Partnership in Future Healthcare
As we look toward the future of AI in healthcare, the most successful models will likely be those that emphasize augmentation rather than replacement. The goal isn’t automated medicine but enhanced human care—systems where AI handles tasks computers excel at while human providers focus on the uniquely human elements of healthcare.
This partnership approach plays to the strengths of both parties: AI excels at pattern recognition, consistent analysis of massive datasets, and tireless monitoring. Humans bring empathy, ethical judgment, contextual understanding, and creative problem-solving to complex cases.
For healthcare organizations considering AI implementation, starting with a clear assessment of current challenges and potential AI solutions is essential. At TechMim, our healthcare AI consultations help identify high-impact applications that align with your specific needs and organizational readiness.
The future of healthcare won’t be delivered by AI alone, nor by humans working without technological assistance. It will emerge from thoughtful integration that amplifies human capabilities while addressing limitations. That journey is well underway, and its potential to transform medicine has never been more promising.
What’s your experience with AI in healthcare? Have you encountered these technologies as a patient or provider? Share your thoughts in the comments below or check out our other AI resources for more insights into this rapidly evolving field.
Frequently Asked Questions
How accurate are AI diagnostic systems compared to human doctors?
AI diagnostic accuracy varies by specialty but often matches or exceeds human specialists in narrow tasks like analyzing specific medical images, with some studies showing 5-15% higher detection rates for certain conditions.
Will AI replace doctors in the future?
No, AI will augment rather than replace healthcare providers, handling routine analysis while physicians focus on complex cases, treatment decisions, and the human elements of care that AI cannot replicate.
How is patient privacy protected when using AI systems?
Modern healthcare AI employs advanced encryption, anonymization techniques, federated learning approaches, and secure computing environments that process data without exposing individual patient information.
What types of healthcare data are most valuable for AI analysis?
Medical imaging, electronic health records, genomic information, and continuous monitoring data from wearable devices provide particularly valuable inputs for healthcare AI applications.
How quickly are new AI healthcare solutions being approved for clinical use?
Regulatory pathways are still evolving, but the FDA now has specialized review tracks for AI/ML medical devices, with some approvals taking 6-18 months compared to years for traditional medical technologies.
Can AI help reduce healthcare costs?
Yes, through earlier disease detection, reduced diagnostic errors, optimized resource allocation, and more targeted treatments—with some systems demonstrating 15-30% cost reductions in specific applications.
Are there AI tools patients can use directly without physician involvement?
Several consumer AI health tools exist for wellness tracking, symptom checking, and mental health support, though most diagnostic and treatment AI applications still require healthcare provider oversight.